If the first black hole picture was a fuzzy doughnut, this one is a thin onion ring.
Scientists have enhanced the depiction of the supermassive black hole at the heart of galaxy M87 using a machine-learning approach, showing a narrower ring of luminous plasma than previously seen.
The Event Horizon Telescope scientists revealed a picture of M87’s black hole in 2019. The image, the first of a black hole, revealed a fuzzy orange ring of whirling gas silhouetted by the dark giant. Despite being based on the same data, the new ring’s thickness is half that of the original, researchers report on April 13 in the Astrophysical Journal Nature.
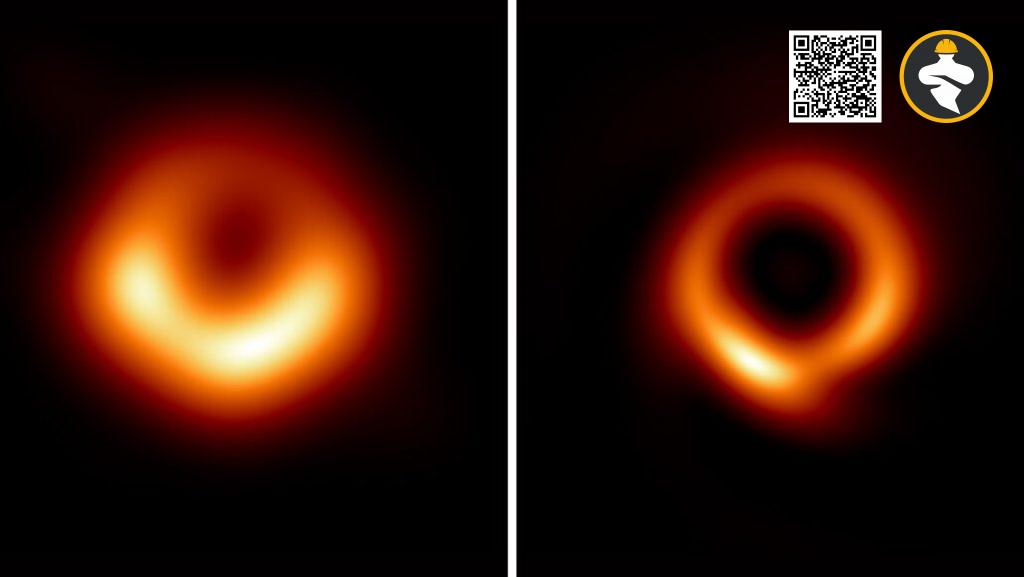
The Event Horizon Telescope (EHT) is a global network of radio telescopes that are synchronized to observe the same target simultaneously. The aim of the EHT is to capture the first-ever image of a black hole’s event horizon – the point of no return where the gravitational pull is so strong that not even light can escape.
However, the EHT method leaves gaps in the data due to the fact that it’s impossible to cover the entire Earth with telescopes. This gap creates a challenge for scientists who want to reconstruct the image of the black hole. In order to fill in the missing information, an algorithm is needed. In the past, this algorithm relied on assumptions such as preferring a smooth picture to fill in the gaps.
However, in 2019, the EHT team released the first-ever image of a black hole’s event horizon using a new algorithm based on machine learning. The algorithm was trained on over 30,000 simulated photographs of stuff whirling around a black hole, which resulted in a clearer image. This approach fills in the gaps in the data using neural networks and image recognition techniques to generate a more accurate image of the black hole.
The success of this new algorithm represents a significant breakthrough in the study of black holes. It has enabled scientists to gain a better understanding of the black hole’s mass and perform enhanced gravity tests and other black hole physics investigations. In the future, this approach may also help scientists study other complex astrophysical phenomena that rely on high-resolution imaging, such as the formation of stars and galaxies.
Reference: Emily Conover@sciencenew.org